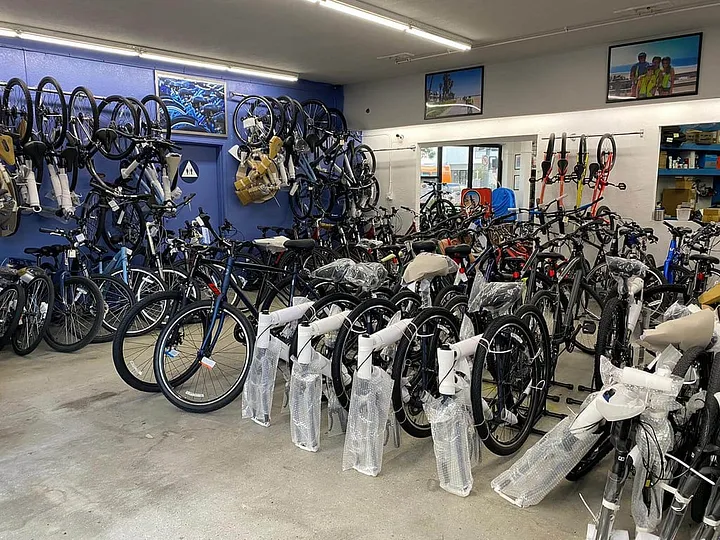
Sprocket Central Pty Ltd, a medium size bikes & cycling accessories organization, is keen to learn more about KPMG’s expertise in its Analytics, Information & Modelling team.
Primarily, Sprocket Central Pty Ltd needs help with its customer and transactions data. The organization has a large dataset relating to its customers, but their team is unsure how to effectively analyze it to help optimize its marketing strategy.
The client has asked our team to:
1. Assess the quality of their data,
2. As well as make recommendations on ways to clean the underlying data, and
3. Mitigate these issues.
Resources provided.
The client provided 3 datasets:
1. Customer Demographic
2. Customer Addresses
3. Transactions data in the past 3 months
My task.
I have been asked to take a look at the datasets received and draft an email identifying the data quality issues and strategies to mitigate these issues.
Tool used.
MS Excel
My Response (Email).
Dear Sir,
Thank you for providing us with the three datasets from Sprocket Central Pty Ltd. The table below highlights the summary statistics from the three datasets received. Please let us know if the figures are not aligned with your understanding.
Table name: Customer Demographic
No. of records: 4,000
Table name: Customer Address
No. of records: 4,003
Table name: Transaction Data
No. of records: 20,000
Notable data quality issues that were encountered and the methods used to mitigate the identified data inconsistencies are as follows. Furthermore, recommendations have been provided to avoid the re- occurrence of data quality issues and improve the accuracy of the underlying data used to drive business decisions.
1. Separating one value into two columns can increase processing time and make it difficult to locate duplicate error. Like in CustomerDemographic table separating the first and last name.
Mitigation -Use only one column for customer name.

2. The gender column in CustomerDemographic has inconsistent entries like F, Femal, Female, M, Male, U.
-I corrected it to allow 3 values only: Female, Male, Unspecified.
The state column in CustomerAddress table has New South Wales, NSW, QLD, VIC and Victoria.
-I changed the values to New South Wales, Queensland, Victoria
Mitigation: -In the user interface of the CustomerDemographic and CustomerAddress forms, use drop down list (add data validation), so users can only select from a list.

3. In DOB column in CustomerDemographic table, we found inaccurate data. One of the dates of birth was set as 1843–12–21. In 2017 that customer would be 174 years. This is inaccurate.
-I changed this wrong value to Others.
Mitigation: -To avoid such error from reoccurring, use a default calendar in the user input form and set a validation between 1 year and 120 years.

4. In CustomerDemographic table some rows were left blank.
-In job title, I replaced the blanks with Others, and replaced the wrong date and the blanks with Others.
In Transactions table I discovered blanks in the following columns: online_order, brand, product_line, product_class, product_size. These values are required to set the price and should not be blank.
-I changed the blanks to Others.
-The number of blank rows is the Transaction table (brand, product_line, product_class, product_size) is 197. This is less than 1% (0.99%) of the 20,000 transactions in the dataset. As such, this error can be ignored, and the data deleted.
Mitigation: -Establish which value is critical and make it required in the user interface. If this value is not provided when filling the form, the form cannot be submitted.
5. In CustomerDemographic table the columns deceased_indicator, default, tenure, have values that have no direct need for our analysis.
In CustomerAddress table the column country has the same value for all the values in the dataset (Australia). No analysis can be carried out. The column property_valuation has values that have no direct need for our analysis.
In Transactions table I removed the product_first_sold_date from the dataset.
-I removed/deleted these columns.
Mitigation: -Only get data that isrelevant to the organization or the analysis.
6. Maintaining two tables for 1 customer can cause updating issues, where 1 file is updated and the other is not. It is better to maintain only one dataset for customer details.
In the process of joining the CustomerDemographic and CustomerAddress tables, 3 rows in CustomerAddress had no matching customer_id in CustomerDemographic table. This is an error.
I also identified this value (5,034) in the customer_id in the Transactions table, that was not in the corresponding column in the master table, CustomerDemographic Table.
- I deleted these values.

In the Transactions table the column list_prize and standard_cost have different formats, but both are currency values.
- I formatted both to currency values, with 2 decimal places.

Mitigation: -Merge the CustomerDemographic and the CustomerAddress tables into 1 table, Customer.
And apply formatting rules especially for the dates, numbers, and currency fields when designing the input forms.
Moving forward, the team will continue with the data cleaning, standardisation and transformation process for the purpose of model analysis. Questions will be raised along the way and assumptions documented. After we have completed this, it would be great to spend some time with your data to ensure that all assumptions are aligned with Sprocket Central’s understanding.
Kind regards,
Anietie Etuk
Sign up today and:
• Get 30% discount.
• Get the full course manual for free.
• Attend a FREE trial class.
• Get a DA certificate after the class.
• Gain access to our DA job database.
Click the link to signup via WhatsApp: wa.link/qswt7e
–
• Location: SkillAhead, 8 Old Aba Road, PH.
• Schedule: Weekday or Weekend.
• Time: Morning or Evening.
• Start Date: 27th March, 2023.
• Duration: 1 month.
• Tools: Power BI, Excel, Google Sheets.
• Certificate: Data Analytics Certificate.
• Fee: N75,000.
• Early Bird: N52,500 (ends on 19th March).
• To register: click this link: wa.link/qswt7e